What I Wish I Knew Before Linking Data
May 15, 2024 | 45:57
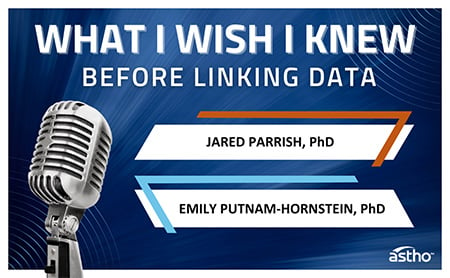
Listen to the interview by pressing play below.
This episode features a conversation between two data linkage experts—Jared Parrish, PhD, MS, and Emily Putnam-Hornstein, PhD—highlighting their lessons learned and sharing recommendations for those seeking to use data linkage projects to examine key public health issues, such as:
- The thought process behind choosing which datasets to link, which linkage tools and methods to use, and how to bring intentionality to these choices when considering a research question.
- The benefits of using data linkage to enhance datasets and build a comprehensive and robust collection of information for new insights.
- Lessons learned for navigating data linkages with important considerations for preparation, analysis, and the uses of data linkage.
Show Notes
Interviewer
- Stephany Strahle, MPH, Maternal and Child Health Contractor, ASTHO
Guests
- Jared Parrish, PhD, Senior Epidemiologist, State of Alaska, DHSS, Division of Public Health
- Emily Putnam-Hornstein, PhD, Distinguished Professor for Children in Need, University of North Carolina at Chapel Hill
Transcript
STEPHANY STRAHLE:
Hello, and welcome, my name is Stephanie Strahle, and I'm the intern with the Family and Child Health Team here at ASTHO. Today, I'm bringing you an interesting conversation about using data linkage projects to explore key public health issues. I'm joined by two wonderful guests who will be sharing their lessons learned for conducting data linkage research.
First, we have Dr. Emily Putnam Hornstein, who is the John A. Tate Distinguished Professor for Children in Need at the University of North Carolina, Chapel Hill. She also maintains appointments as a Distinguished Scholar at the University of Southern California, where she serves as the Faculty Co-Director of the Children's Data Network.
Dr. Putnam Hornstein is a Research Specialist with the California Child Welfare Indicators Project at UC Berkeley, and for nearly two decades, she has partnered with public health agencies including the California Department of Social Services and California's Health and Human Services Agency to carry out applied research to inform child welfare policy and practice.
And secondly, we have Dr. Jared Parrish, who is the Senior Maternal and Child Health Epidemiologist at the Alaska Division of Public Health.
He also operates Parrish Analytics and Epidemiology Consulting, and his research interests focus on child and adolescent injuries, administrative data linkages, longitudinal data analysis, and incorporating novel methods for applied surveillance with an emphasis on improving timeliness, efficacy, and the utility of data that lead to prevention.
So hello, Jared and hello, Emily. I'm so excited to be here with you today. I guess I'm going to just go ahead and dive right in with the first question. For those unfamiliar with data linkage, can you give a bit of a brief overview of what data linkage is and what this process aims to do?
EMILY PUTNAM-HORNSTEIN:
Sure, I'll start. This is Emily. Thanks, Stephanie, so much for facilitating today's conversation. In the public sector, we capture all sorts of information about individuals we serve through various programs. Data linkage is really just the process of trying to connect the records across those different data systems so we have a more complete picture of who people are, what services they received over time, what outcomes we observed, and also who shows up in certain data systems and not others. So, my kind of work started with trying to answer a question around which children entered the child protection or child welfare system. We could see a lot of data in the child protection system about those kiddos, but we didn't have much information that allowed us to make comparisons to broader populations. And so, we did some linkage child welfare to birth data.
JARED PARRISH:
No, I agree with Emily. When I think about what data linkage is and what the intent and purpose is it's really what we all want when we're thinking about like our own medical histories when we go from doctor 1 to doctor 2 to doctor 3, it's why I fill out this thing every single time, why aren't these all speaking together? And we're just trying to do this on a larger scale to try to connect information so that we can learn More fully from what we would learn from any single source.
STRAHLE:
Thank you for that. Um, I will go on into the second question. So, going back to the first time you ever conducted linkages, or what got you started in linking data, what did you first decide to link data to, and what sources kind of did you land on, and can you touch on why data linkage was such a useful tool for your respective research question?
PARRISH:
Stephanie, when I was thinking about this question, it made me laugh a little bit, because over 16 years ago, when I first came up to Alaska, I was coming up as a CDC fellow pretty recent out of my master's program, thought I knew everything, and I was like, sure, I'll go link data, and the idea was to come up and try to do, or bring a public health perspective to child maltreatment surveillance, so that public health could try to do some intervention on that, and I was so naive. And I came up, and I failed miserably on my first attempt. I approached it as I'm going to grab law enforcement data, I'm going to get vital records data, I'm going to get Medicaid data, I'm going to get child death review data, I'm going to get all the data from the child advocacy centers, and I'm going to get our child welfare data, and I'm just going to bring it all together, and it's going to be magical. And I got all the data, and then when I started trying to connect all those data pieces together, I realized I was in way over my head. And I didn't know what I was doing. But I felt coming straight out of school as a master's student that I had to prove myself and that was somehow not okay to go ask for help because that would show that I didn't know something and I that's where I made a huge mistake there, instead of going and just asking for help I tried to connect these things and made multiple mistakes along the way. I remember the first time I ever did it, I concatenated all these identifiers into one cell and then merged them together and did that on multiple iterations and multiple steps. And couldn't make heads or tails out of it and I didn't know what my population was when I was connecting records. Now I know whether it was an inner join or an outer join or a left join or a right join. And I've floundered with that. From that experience of floundering, I realized some really important things that we'll get to later on in this podcast, but my initial temp was try to bring all these data sources together into one common pool that then I could analyze from it, and so that was my experience for my first time.
PUTNAM-HORNSTEIN:
So, I think I was a little bit less ambitious than Jared. The first time I tried to link data which I guess was a good thing. I was a doctoral student and for my dissertation, I was interested in answering the question. how many children who had been reported for alleged abuse or neglect ended up dying in the first five years of life. So, I was trying first and foremost to connect statewide administrative child protection records, to vital death records actually benefited from the use of an open-source linkage tool that was put out by the CDC link plus, which was originally Designed and released to help states connect cancer registries and vital death record registries. So, I used that as my tool and learned a ton along the way including all sorts of little things that can go wrong with your linkage. But that was my first foray into this world.
STRAHLE:
Thank you, for both of your little insights into the beginning of it for your journeys. I think you've already touched on the fact that data linkage as a process is pretty complex and there are some nuances that researchers should consider before they go into a data linkage project, and so this leads to me to my next question. What and why do you think are the most critical things to do prior to linking to another source?
PARRISH:
I'll start, Emily. What's first I think is important to remember is that a lot of people have been linking data for a long time. And. Connecting records and sources. And it's interesting when you start talking to a lot of people that have been linking data for a long time or went through this experience almost all of them have some sort of iterative learning experience. And so, I think going into linkage, knowing that you're going to learn some things and be okay with that and have some uncomfortableness is going to be a good thing. But with respect to the linkage component, for me, what I've come down to is knowing your “why” and knowing your “who” are two critical things that need to be started, need to know, before you even start your linkages, because know why you're linking those data. What do you expect to learn by bringing those sources together? What's your why? And Emily had a really good why, and I didn't. I had a poor why when I first started. And knowing your who. Emily had a really good knowing her who. They wanted to expand to this statewide birth population, and I didn't know my who. I was just linking all these data together to think that I was somehow going to know who my who was going to be. And that really comes to knowing who your population denominator is going to be, who you're going to be talking about, and what you expect to learn. Those are some things that I think are really critical before you start a linkage project because it just sets you up for success from the get-go as opposed to what my first experience was. I don't know, Emily, do you have thoughts?
PUTNAM-HORNSTEIN:
No, I really like the way that you framed that in terms of knowing your who and the population and the why are you linking, what is the question you're answering. I think the only other thing that I would add is there is a lot of pre linkage work that will probably save you time in the long run. So, on my team we always have a process where we have a series of data hygiene checks where we're really vetting that the data sources, we are trying to link are passing the gut check. We're seeing the right years. We've got complete information in the fields where we were expecting it to appear. We know exactly which identifiers are present, where they're missing. We've done cleanups. A perfect example is that when we do work to link up vital birth records with child protection records, we will see a number of records in our child protection system and in our birth data where the name simply shows up as baby girl or baby boy because at the time the record was generated a name had not yet been assigned or at the time the call of potential abuse or neglect came in the name of the child was not known, and if you don't figure out how to clean that up and address that on the front end, on the back end, you're dealing with a lot of Clearly errant record linkages where we're matching up baby girl and baby girl, even though there is absolutely no basis for thinking that is the same person. So I think that's the only other thing I would add is don't underestimate the good investment that is cleaning up and really getting to know your data sources before you go forward in trying to link them together.
PARRISH:
Emily, you bring up such a great point, and I am going to steal and take that phrase, data hygiene. That is very explicit, I always think of it like data cleaning, and then doing some data harmonization that are such techie terms. But you can really think this idea of cleaning it up, scrubbing it down, and I agree with you. I think that is a critical step that goes into that, and it's quite interesting too, how I find that it ends up, I learned things about my population when I go through that process about, Oh, wow there's a lot more uncleanliness or dirtiness in my data with different racial groups because maybe they don't know how to spell names or they make inferences about different groups which could have this biased result on my linkage results at the end that I'd be trying to figure out. So I, I think that's a really good point about it and I love this term data hygiene.
STRAHLE:
we'll coin that and make that even the title of this
PUTNAM-HORNSTEIN:
I'm sure I probably stole that from someone else, but uh, but I'll claim it, Jared, and you can use it.
PARRISH:
That's great.
STRAHLE:
In addition to thinking about, the who and the data hygiene of your data sources what goes into the thought process as well of choosing tools or methods for your linkage?
PUTNAM-HORNSTEIN:
I'm happy to start because the tools that I have been using and my team has have really evolved over time. As I mentioned, my, my first ever linkage was simply using the CDC Link Plus tool which, by the way, is amazing, so I'm really glad that's still out there and it definitely met my needs at that time.
But there were also some significant limitations so for example, in that and some of the other linkage tools that are out there there's a kind of a ceiling to how many records you can read in. In batch mode at a time. And so given that most of my work in California involves millions of records, sometimes tens of millions of records that was a real challenge as the linkages grew.
The other challenge was that because that system and many other linkage programs are designed to be a little bit turnkey for the user, There was no opportunity to train the algorithm that was being used to determine which record pairs were a potential match and the weights that should be attached to the probability of that match.
And so started there, but over time, what we have done is to develop our own code. for training an algorithm that is really customized to the sources of data we're using in California. So there, I don't, I certainly do not want to present this as you always need to have a customized tool.
PUTNAM-HORNSTEIN:
We're using a lot of other open source packages and technologies that are out there. But I think, If you are embarking on a multi year, longer term project really making sure that you have an algorithm that reflects the data sources you're trying to link is probably a good strategy.
PARRISH:
Emily, when you were talking there, I was thinking one thing that, that I think is important to recognize that maybe we've missed on is that there's these two main kind of themes within linkage, deterministic and probabilistic. And when, what we're really talking about is this need for probabilistic record linkage where we don't have any direct identifiers that we can say with 100 percent certainty that individual A, in record set A is the same as individual A in record set B.
And so we use these partial identifiers like names, dates of birth and sex or gender or region or whatever. And we try to use those combinations of factors to help us identify the probability that this individual in record set A is the same individual in record set B. And so these tools help us try to isolate and reduce down those probabilities.
PARRISH:
And there's this degree of manual review that we do. That is sometimes required and often required when you're doing these probabilistic record linkages. And so through the evolution of data science coming into public health and being more accessible with our computing power, you've seen a lot more of these machine learning type algorithms to try to really address This manual review process that is subject to error when you actually involve humans.
And so if you can train computers to do these types of things, you can actually get more consistent results. And so this evolution that Emily was talking about, my evolution was I started with Microsoft access and just trying to develop different models for that because that was the tool that I had available to me in a health department.
And that is a big limiting factor that a lot of people across the country face when they work inside a health department is their IT structure really doesn't allow them to leverage these innovative tools that are available to them. But when I was really exposed to the degree of of importance of weighting different variables that you're using was using frill, it was fine-grained record, linkage. I can't remember the acronym, but it was developed by a researcher out of Emory. And what was it was this job of the JavaScript based platform. And what was great about it is that you could control how much weight and which type of algorithm you wanted to use for each individual factor, like first name, last name, date of birth, and you could in real time see the influence of that change on your probability scores.
And so that was a powerful tool. And it's a It's still out there. And sometimes I even go and grab that and use it because it's so quick to see how quick I can change that or how that would influence my linkages. And because I'm not faced in Alaska with millions of records I'm faced with thousands of records.
I've never ran into this issue of needing data a tool that can handle large data sets. So I've used R a lot in my record linkages because I have a lot of flexibility in the coding over that. And there's two great packages in R. The record linkage package and the fast link packages. And there's some really great linkages now in Python for doing record linkages that allow this probability record linkage to occur.
But I think the take home here is that you have to know your why. And know your who because when you look at what population you're going to bring together that can influence which tool you might use. Because if you have direct identifiers, like you have social security number in both places, there might be a little bit of error to that.
But you might be able to use a, a different tool than say, something that has very limited identifiable data where you need some big probabilistic capabilities.
PUTNAM-HORNSTEIN:
Jared, can I just jump in by saying that is a very good point, and I'm glad that you remember to define deterministic versus probabilistic.
We probably should have started with that and to just add to that, I think that the other thing that's important for different folks to, to think about is the kind of the frequency with which the linkages need to be done, right? So are we talking about a a use case or a why?
Where you almost need the kind of the data linked and integrated for operational kind of real time use cases where it needs to be done very frequently. Or are we talking about linking data? For let's say a policy report that needs to be prepared for your state legislature once a year and so the trade offs and the thought that goes into which tools will be fit for services is going to depend on that frequency piece as well.
STRAHLE:
These are great points. You're talking about Waiting and stuff like that. I just want to pivot toward to like assessing the quality of linkages. What are some things you worry about when it comes to the quality of your linkages when you're conducting them?
PARRISH:
This is an area that can drive a Type A person insane. Worrying about the quality of data linkages and what can become really difficult is that often you don't have a gold standard from which you're operating on. And so you can become worried about, How much checking I need to do on my data, and how do I check it in a systematic way that helps me feel comfortable with accepting a certain amount of error?
And how do I quantify that error? These are all questions that, that I get faced with every time I do a linkage. And the first thing I've had to teach myself is that, There's already a lot of error in these data to begin with, and so there's going to be error at the end of this. I just need to be able to understand what that error is, and how that may influence my results.
And what I really initially start looking at is what's my intent and my purpose behind this? If I'm just doing public health surveillance, And that's all I'm going to be doing. Emily's talking like I'm putting out an annual report, and the frequency of it is simply annually. But I just wanted to be able to determine if trends are going up.
Or if they're going down or staying stable. And that's my primary purpose behind that. I am going to likely do a degree of quality checks on my data. Feel comfortable with a threshold where I cut off and accept cases that I know have, that I've got. Cases that actually shouldn't be linked and then ones that I'm rejecting.
And I'm going to do that the same way each time over year after year. So that I can feel comfortable in what my trends are. And so when I Conducting quality checks on my linkages. I worry about, I haven't defined my Y very well. And so that I'll spend a lot of time spinning my wheels, going down paths of trying to determine if John Smith, who was born in the year 1950, is the same or different John Smith that was born in 1950 in this other data set that has 20 of those John Smiths.
And how to determine which John Smith that goes with. That's where I could get myself into problems is getting focused on that. I don't know, Emily, if you have thoughts on this.
PUTNAM-HORNSTEIN:
Yeah. I guess I was trying to think about it less from the technical perspective and more from the specific question that you're trying to answer and therefore what the missed linkages or the error in your linkages potentially represent.
I don't know that I have a perfect example, but Jared, I was thinking about when. When we linked up child welfare and educational records in California, we were able to match about 80 percent of students who were enrolled in K through 12 to a to a birth record. And then we could also look at child welfare data.
So the question is always what about the 20 percent of students you were not able to match to a birth record or another source. What do they represent? And we were able to do some checks to confirm who we thought we weren't linking and describe what questions we could or couldn't answer based on that.
But then there are other questions where you're actually trying to develop let's say, some kind of cumulative incidence number. And so every single record that don't link, you're assuming that a person didn't have an encounter with some other system. So to be very specific, when we've linked birth data to child welfare data to try to figure out how many kids, let's say, get reported and investigated by age five.
If we link and find that about that's true for about 15 percent of kids, We're assuming that we're accurate and the other 85 percent of kids truly didn't have child welfare involvement. But if there are major kind of out migration shifts for certain groups, or if the quality of the data really varies across either counties or demographic groups the implications of kind of those missed matches means something that's very core to the question we're trying to answer.
I don't know if that's actually a, I may have come up with bad examples there, but but in my rambling, I hope that, the key thing I think is to think about. For the records we didn't match, or the errors that potentially exist, what are the implications for the findings that we think we are able to present from these data?
PARRISH:
Emily, that's a great point. It reminded me of that that paper that I wrote years ago on where I talked about the non linkage assumption because in Alaska when I was doing that longitudinal follow up of my population, I was able to link to a source that was able to account for out of state e migration and I was able to compare what would be the estimate if I didn't account for that and what would be the estimate if I assumed or if I was able to account for that, if I had this non linkage assumption.
I think that's a great point of trying to spend some time understanding what the impact is of those that don't link to the administrative source, especially when you're doing longitudinal follow up of an individual over time. Those are great points. The this question was like, what are things you worry about when conducting linkage?
It's it's interesting when you go and you talk to like the people who have blazed the trails before us and I think of the Russ Kirby's of the world or the Milk Coddle Chucks of the world that have been doing data linkages for a long time. And when you hear And then listen to them talk about their linkage journey on that.
They spend a lot of time actually talking about what Emily talked about before. It's like knowing your data really well, doing your data cleaning on this. Because then that sets you up for having some idea of what could be impacting your linkage probabilities. Like I know there's certain data sources that we have I've been, even our our vital records data for a while had a limitation on the character string that could be made for, put in for names, and our Polynesian names are usually really long, and so there would be, like, these truncations that would occur that I didn't even know were happening, And that could impact the quality of my linkage for a given population, which then if I go to compare them, could result in some biased estimates.
So those are the things that I worry about, is that somehow I've got differential linkage quality by groups that I want to compare, that then results in me making an inappropriate inference.
STRAHLE:
I will go on to the next question. What have these linkages been able to Give you insight into your research questions that you otherwise wouldn't have gathered.
PARRISH:
Stephanie, thank you. This is the opportunity I have to a little bit maybe redeem myself from the beginning of this thing where I failed miserably. First of all, I've had to learn that it's okay to make mistakes along the way, and that's an okay thing to do.
And what happens in that journey is that you find some little golden nuggets along the way. And so from a public health perspective, I was able to land in on linking the PRAMS data. With a variety of other administrative data sources and for those not familiar with PRAMS it's the Pregnancy Risk Assessment Monitoring System Survey and it's a sample of live births each year that ask about the pre birth, and shortly after delivery experiences.
And I was able to integrate that that survey response or an epidemiological source with these administrative data that alone the PRAMS data is great for giving annual prevalence estimates. But linked with child welfare data, I was able to actually start understanding connections between pre birth experiences and the probability of child welfare involvement later on in life.
And so that connection of understanding the context of something that's occurring before an outcome is happening gives really strong public health gives good information to help us build our public health model on, which is what are, what's the population risk and protective factors that we can try to intervene on to improve the lives of these children moving forward.
And so for me, it was really about making these mistakes, identifying a data source that was actually attainable and reasonable and consistent that I could use on a regular basis. And that by connecting. And Emily and I this is why we know each other is because we both were looking at child maltreatment as this outcome that we were interested in and what were these early birth factors that we could be that potentially mutable that we could address that if we only looked at child welfare data, we wouldn't be able to understand that.
And if we only looked at our vital record, our birth records or our PRAMS data, we wouldn't be able to understand that connection. So by bringing those two together, we're able to learn something new. Okay.
PUTNAM-HORNSTEIN:
And for the purposes of the recording, I'm also going to try tackling a question that I feel like I flubbed because Jared made the answer at first.
Think there's that question of, at its simplest, what is record linkage? And for the longest time, I would always open all of my presentations by one of my favorite quotes. It's from the American Journal of Public Health, 1946, Dunn, who described record linkage as, Each person in the world creates a book of life.
The book starts with birth and ends with death. Its pages are made up of the records of the principal events in life. Record linkage is the name given to the process of assembling the pages of this book into volumes, and I think that's just a really nice and simple way of describing it. It's hard to beat that, but I will also add that another way to think about this from a data perspective is you've got an Excel spreadsheet, and you know who your population is because you've got a lot of rows.
But you may not have that many columns in that spreadsheet helping you understand who those people are or what those records represent. And so with data linkage, often the number of rows is staying the same, but you're able to pull in that many more columns to help understand the population you're interested in.
PARRISH:
So Stephanie, I don't know if we answered your question, but we spoke to what we felt. Emily, I need to print that out and put up on my wall, because you're right, That is such the important and it made me instantly go back to my mom who's huge into genealogy and does all this time looking through all these records and trying to connect people and their family histories through time.
And that's just data linkage at the individual level where you're trying to say, Oh, is this person actually this person who's connected to this father or mother? So there's people doing all this data linkage at individual level. At, and we're just doing it at the population level.
And I think it was summarized very well that way. So thanks for sharing that.
STRAHLE:
I might ask you for that quote after and then put in the description. It's a little great tidbit. So I'll move on to the next question of if you could go back in time to the first day you decided to do linkages, what would you do differently?
PARRISH:
I'll start Emily. I, sure. Wouldn't try to link all the data sources that I tried to link. I was impressed that I was able to get all those data sources together. So I felt good about that. But I would not be as ambitious. I would start small and be specific. And especially from being linkages within the health department.
And even though in the health department, there's a big push for data modernization. That's just a new name for something that's been trying to be improved for a long time. We will continue to see ourselves try to modernize and improve our data sources. But the, within the health department, When you can link two data sources together and you have a clear purpose for it and you're very specific and you have a question and you show the utility of it and the impact of what it's worth is, it opens up doors to increase your data linkages and your data sharing.
And so for me, what I would have done is I would have started small and I would have been a lot more specific to show its utility.
PUTNAM-HORNSTEIN:
Yeah, so that's a great question. And I think if I had to go back and do something different I would probably think about structuring some of the data use agreements a little bit differently.
When I first started developing the data use agreements I was both asking for all of the personally identifiable information or the confidential data that was needed to establish those person level linkages across various data sources, and I was simultaneously asking for a lot of analytic or service information that I would then use as part of my analysis.
And I was always very clear that we adhered to what is sometimes referred to as the separation principle. So the process by which Members of my team were handling the confidential data and the security and how that data was handled and used was very different than once we got to the analytic stage on our team.
We have data scientists who are working in very secure settings. They are only working with the confidential data. They're establishing the linkage. And then when we get to the analysis phase, Researchers are only seeing an anonymized kind of file but they have additional fields and service information and kind of we've created a linked data set for analysis.
And I think that in my original data use agreements, I didn't make those distinctions carefully enough. And so we had to jump through a lot of hoops because understandably, lawyers and others were really unclear as to why researchers maybe needed names or social security numbers. And so I think that being really clear that there are often two parts to these projects, there is the linkage part and that is a precondition and yet also something Separate from the analytics that follow is helpful to getting these agreements set up.
PARRISH:
Okay. Emily, you just opened up a can of worms there. You mentioned data use agreements. I think we should spend a little time on this because there is. There is the legal relationship, and then there's the personal relationship that is required for data linkages. And I almost think the personal relationship is more important at the beginning of it to institutionalize your linkage.
And that process, I remember when I first started this, I would go and be like, Okay, I want to link juvenile justice data in. And how do I, who do I even start a conversation with to to link those data or get access to those data. And so I started these conversations. I would have amazing conversations with people who had absolutely no authority or power to help me.
And they were excited about it and I felt really good about it and nothing would happen. And I would get frustrated and frustrated. And so I learned that when I needed to create that personal relationship that I would have these conversations with the person who let me in the door and then I wouldn't try to sell to them because they were, I would learn to say, how can you help?
How can this person help me navigate in their system who I need to talk to and how I need to get there to establish that legal relationship between it? And that's a process and a journey and takes a lot of time. I underestimated how much time needs to be invested into developing personal relationships, navigating systems, and establishing something that, that could be institutionalized that if I leave, could continue on.
That that's why you institutionalize it. When I first came into this role, there were data linkages that had happened before me, but they were all based on that personal relationship. And so when I went to try to request those data, they're like, I don't know who you are, and I don't know what your process is for this.
And I agree with Emily that being really thoughtful and pragmatic about the confidential the PII that you're using the personal identifiable information that you're going to use. I have agreements with our department of education where the processes where we link, we only share the PII data to create the linkages.
And then among those that link, I request back based on the student ID, the information that is consistent with that. And that relationship is really nice because it, I only obtain. What I link and what I have the necessary data for that, that goes into this research analytic file. But that was a process that was learned along the way.
And so I agree, I would probably, if doing this over again, I would recognize, Okay, how do I navigate having conversations with people? How do I sell this a little bit? And then, how do I set up these agreements that can live outside of me? And that can adjust and be a little bit It can be updated fairly easily without having to go through this whole long process again.
And yeah you spurred a bunch of thoughts, Emily.
PUTNAM-HORNSTEIN:
Yeah. And I'm going to, I'm going to piggyback on that because I do think it's an interesting question. I feel like I'm at UNC Chapel Hill I'm in a university environment and and I struggle because on the one hand a lot of the data that I the only agency that I currently have access to was developed or was probably available to me, Jared, because I took the time to develop the personal relationships to be a trusted partner to a number of county and state agencies, and I again, on the one hand, I think it's unfortunate that we haven't made more Linked curated coded data sets available to researchers broadly because I do believe that these are data that can and should inform all sorts of public policy discussions.
On the other hand, in my old age, I have also become increasingly aware of Just how many people are very comfortable just asking for data and never doing the kind of necessary back and forth about not just the why the person is asking for the data and what question they think is important, but what question is actually important to the agency that's going to have to take the time to bring the lawyers to the table, set up the data sharing agreement, and and so I, again, I am all for finding ways that we can do a better job making those data available.
But I'm also increasingly aware that having access to that data comes with a high degree of responsibility as well, because these are not Just data where it's some hopefully representative national sample where people answered some survey questions. These are going to potentially have direct consequences for the agency in terms of how people perceive its functionality and the outcomes it's producing for Individuals.
And so if you have a researcher who comes in and thinks they understand different fields or thinks that they understand who's missing from a linkage or not, and they just completely get that wrong. There are real world consequences. To that errant research. It's not just that it maybe gets published in a poor journal.
Potentially it has much more significant ramifications. So that's my, I guess my add on slash evolving thinking on the interpersonal piece of this. I really like projects where there are long term relationships and the researchers are working hand in glove with an agent. To figure out what are those important questions that have policy relevance and can be answered with the data we have.
PARRISH:
All right. I'm going to build a little bit more on that one too. I think we struck a chord here. The from my state hat working as a state individual who has data sets around that has researchers requesting data.
And I serve on our scientific review committee where we have these requests that are coming in, and there's always requested and data stewards in the state always sit at this crossroads is they don't have enough time to do their own job, let alone Put together and curate data for researchers to be able to analyze that may or may not benefit the state that will likely get published in some peer reviewed journal, but will likely not get translated down into information that we can do to improve the lives of the people were responsible to serve.
So we're faced with this challenge of trying to sift through all of these requests to say, where are we going to put time and resources and energy into? And I always felt like. When I watched Emily building this thing in California, I was like, man, if I could replicate myself as Emily to build these relationships, this trusted partner, maybe things would go a little bit smoother.
But she put, she just made a great point is that she invested in this time to become a trusted partner. And the thing that can lose trust quicker than anything else is operating on assumptions. And when a state entity sees that someone's operating on assumptions and if they flounder at all.
Not only do you burn the bridge for you as an individual, you burn it for your institution likely. And that's a big task. If you can go into an entity to show how you're going to help them with their work, as opposed to add to their work, you're going to gain a long ways on that.
STRAHLE:
But I'm sensing a theme of deep and thoughtful intentionality when approaching data linkage projects And I'd love if you could close with some final words of wisdom when thinking about that intentionality piece.
PUTNAM-HORNSTEIN:
I guess Data linkage should always be a means to an end, not an effort in data linkage for the sake of data linkage. And so I think clearly articulating what that end is is helpful kind of across the board, covering everything that we talked about from how you establish your data sharing agreement.
To the specific records that you're starting with to the establishment of a partnership with those from whom the data are being received, that helps to really answer important questions.
PARRISH:
And I'll just basically echo what Emily is saying. And that my, my intentionality is recognizing that I'm bringing together record sources to try to help improve the lives of people, of individuals.
And this idea of bringing a mass amount of information together is really about individuals and their lives and trying to improve that so that they can be as healthy and as successful as possible.
The one thing I think is important to, to remember is that A lot of federal entities, like the Centers for Disease Control, they're investing in data modernization, and I mentioned that before, and that's really about bringing data together and allowing these systems to talk and communicate more effectively together, so that these big, huge efforts of these big data linkages maybe won't become as big and huge as they might be.
But while that's occurring, there's a lot of things that you can do for linking data together to answer specific questions to help the lives of people now. I don't think, don't get contribute to this modernization effort. Get your data working together. But I think there's also a lot of power in doing proof of concept data linkage projects that are very specific and focused to show utility.
That can help improve things now. And the CDC has funded AASHTO to put together resources for those that want to link PRAMS data. And that's where I focus is linking PRAMS data, survey data with administrative data. But I also know that the CSTE fellowship has entity has a lot of good resources on data linkages from a public health perspective.
And the, there's a lot of of amazing people building these machine learning type models now that are out there and that requires a steeper learning curve to make sure that you know how to do those, but I think that's the way of the future, especially for really large data sets.
PUTNAM-HORNSTEIN:
What I'm about to say may contradict something I said before, but when we first launched the Children's Data Network to begin doing some wide scale linkages in California, we had a kickoff meeting and we looked at some of the kind of countries that were way out ahead of us in terms of having integrated linked data for researchers to use. And my my favorite story is that I reached out to a colleague from Denmark.
His name was Peter Falison and and, he reflects on how for the first few conversations we had. He had absolutely no idea what I was talking about because he didn't understand what this thing of linked data was, and he finally said Emily, in Denmark, we just call it data. So I close with that because I would love to, to see us in the U.S. Get to a place where podcasts such as this are dated because we don't need to talk about link data. We just have data as they do in Denmark and a bunch of other places.
PARRISH:
Emily, I'm going to follow up with that. That is such a great point. When you go and talk to other countries that have this, they're really confused at why we have all this effort.
It's so true. If you guys don't mind, I might also just add about a year ago, I was presenting some results from these linked data where we're showing the relationship of factors before a kid's even born and outcomes early on in childhood. And after the presentation, an individual came up to me and I could tell the individual wanted to talk to me but was a little bit nervous and so I engaged them.
And they started sharing with me that these models that I was presenting was their life. And they were trying to understand, like, how could this statistical model be showing what their life was? And they were just blown away by it. And that was the moment when, and this was just like last year, where I really started shifting from population based data can teach us everything.
It's really, these are people's lives. And when the data comes together, and it's, and we bring more information together, people whose lives that we're describing see it, see themselves in these data. And we need to make sure that their voice is a part of the story that we're telling. And so having some qualitative lived experiences is really powerful to bring together with these big linked data sets because we are describing people's lives.
It is real experiences for a lot of people. And when they start sharing the story and seeing the power in the data, it just tells that story a little bit more powerful, and I'll stop there.
STRAHLE:
No, that was great, Jared. Thank you. Thank you both for being here today. I am so excited to have had this. Opportunity to have this conversation. Data linkage can really open up so much opportunity to gain deeper insights into public health issues, especially in the realm of maternal and child health. But that's all we have for you today.
Remember, for all of your public health information, please visit astho.org.